Post By: Safer / 4 min read
At Thorn, we want to make online spaces safer. As part of the trust and safety ecosystem, we understand that the challenge of protecting users from harmful content, especially child sexual exploitation (CSE), has intensified. Over the last year, we’ve vigorously studied these emerging threats, so that we can develop technology that safeguards children and digital communities. The result is a significant expansion of our Safer solution, now including text detection for CSE.
Safer Predict is a state-of-the-art AI-driven solution designed specifically to combat child sexual abuse material (CSAM) and text-based CSE. Leveraging machine learning, Safer Predict analyzes text conversations in a variety of contexts such as direct messages, forums, and posts, it identifies potential threats such as bad actors producing, selling, and trading CSAM, sextortion threats, and other forms of sexual harm to minors.
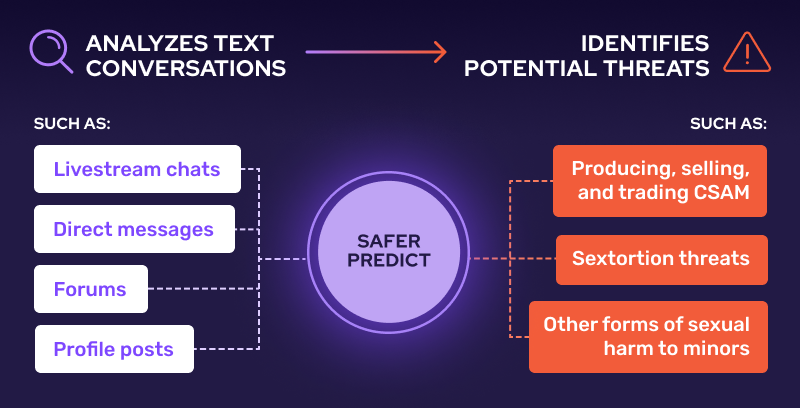
Before launching our text classifier, we completed a comprehensive beta period with trusted partners to ensure the product was robust enough to meet platform needs. In this blog post, we share our findings and insights gained during this crucial testing phase.
Real-World Impact and Success Stories
During beta for Safer Predict’s CSE text detection, we demonstrated significant success in identifying egregious content, which enabled trust and safety teams to submit high-priority cases to NCMEC. Testimonials from beta partners underscored its effectiveness in streamlining moderation workflows and enhancing the overall safety of their digital environments. Our main successes include:
- Report Escalation: In the short, 4-week period, one partner escalated two reports to NCMEC: one that involved a user grooming a child, and another that involved a user creating CSAM of his girlfriend's daughter.
- Added context: Our partners mentioned that an indicator for the presence of a minor would help them prioritize the results faster. We added a label called “has minor,” which flags text sequences where a minor is unambiguously being referenced (specific age, mention of being underage, etc.). This label is useful when stacked with other labels, such as child access or sextortion to help increase actionability for moderators.
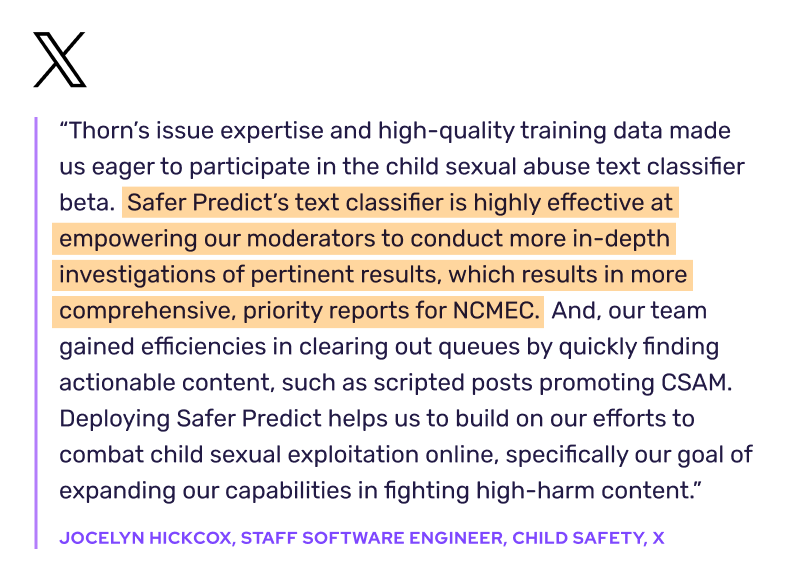
- Detection Optimization: Using real data examples and feedback from our partners, we retrained our model in a matter of days to reduce noise for moderators.
- Pattern identification: The model was excellent at “linking” abuse, meaning, platforms could combine the score of the parent post with the score of a reply to differentiate accusations vs. legitimate concerns.
- Noise reduction: The model revealed to platforms the frequency of both spam and role playing, which were louder than anticipated. The results help trust and safety teams reduce noise so they can focus on detecting and reporting true abuse. The “CSA discussions” label helps streamline child safety workflows by identifying text sequences that discuss CSAM out of outrage, humor, satire, or sarcasm.
These examples highlight the tool's ability to identify and prioritize actionable content, supporting high-quality reports for NCMEC and actional information for law enforcement.
Discovering Common Use Cases
At the conclusion of the beta period, we found common use cases to include:
- Proactive Investigation: Content moderators can run the model over large amounts of text and review high confidence predictions for high priority categories every few days to conduct more in-depth investigations.
- Reactive Triaging: Prioritizing user reported messages for content moderators so they could quickly find and report actionable content as well as being able to identify the most actionable bits of information to include in high priority NCMEC reports.
- Automation: Platforms may be comfortable semi-automating workflows. In one specific example, a partner considered automatically decreasing the public visibility of flagged content on their platform, based on the label and confidence score while waiting for manual review to confirm whether the content indeed violated policies.
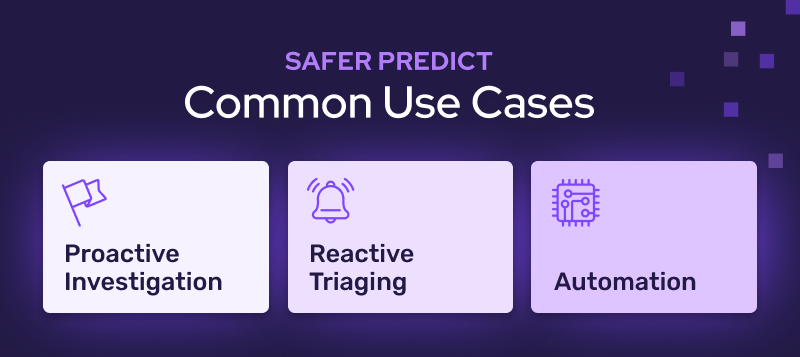
These practices can help platforms become more calculated in their review and reporting of content, ultimately reducing exposure for moderators and accelerating the time from identification to takedown.
Looking ahead
Safer Predict represents more than just a technological innovation; it's a pivotal solution in the ongoing battle against online child exploitation. By leveraging AI to proactively detect and mitigate risks, platforms can uphold their commitment to user safety and regulatory compliance while maintaining a vibrant online community.
As an ecosystem, we know that offender tactics are continuously evolving. Thorn's data science, research and engineering teams are continually updating our classifiers to keep up with the ever shifting landscape so that platforms can stay ahead.
As we move forward, the collaboration between Thorn and platform partners remains essential in refining and advancing these technologies to counter emerging threats effectively. Together, we can create a safer internet where children and digital communities are protected from harm.
For more information on how Safer Predict can enhance your platform's safety measures, we invite you to explore our upcoming webinar or contact our team directly. Let's work together to build a safer digital world for all.