An incomprehensible number of images and videos of children suffering some of the worst abuses imaginable are being shared online every day. Reports of this abuse, made to the National Center for Missing and Exploited Children (NCMEC) in 2023 amount to almost 200 files a minute. Child sexual abuse material (CSAM) is a pervasive problem online, and every platform with an upload button or that provides access to a generative AI model is at risk of being misused to further sexual harms against children.
Multiple CSAM detection methods are needed for comprehensive coverage
Not all CSAM content can be uncovered using a single approach. Therefore, a multipronged approach is needed to provide robust and comprehensive CSAM detection. While still challenging, content that has already been detected and verified can be found reliably by using perceptual hashing tools like Microsoft’s PhotoDNA, Meta’s PDQ and SaferHash. However, identifying content that is not already known to law enforcement represents a larger challenge. This is where artificial intelligence (AI), can provide a lifeline to content moderation teams.
In an ecosystem where the capacity of trust and safety teams is often overwhelmed, having tools that are both reliable and scalable is essential. There are many approaches to detecting CSAM, however, purpose-built hash matching and AI in the form of machine learning classification models (a.k.a. classifiers) are the most robust and scalable solutions available to find CSAM, so that it can be removed and reported.
Understanding CSAM: Legal definition vs. reality
The legal definition of CSAM is straightforward. Moderating this content is more nuanced. A recent example that illustrates this well came from Bluesky. A sexualized image of a dragon that shared visual similarities with a human child was shared. Their trust and safety team had to determine if the content violated their community guidelines (which makes no mention of anthropomorphic dragons). In this instance, Bluesky’s content moderators also wouldn’t find much guidance by referencing the definition of CSAM from the U.S. Department of Justice:
“any visual depiction of sexually explicit conduct involving a person less than 18 years old.”*
*from the U.S. Department of Justice, which also notes that while references to child pornography still appear in federal statutes, CSAM is the preferred term.
For a content moderator, determining what is violative and needs to be escalated can be more complex. There are clear cut cases involving young children and blatant sexual abuse, but there are also many edge cases where a quick determination becomes more difficult. When reviewing potential content violations, moderators must take a myriad of factors into consideration:
- The context
- Age of the victim
- Adversarial manipulations to the content
- The evolution of technology
All of these factors and more can add to the background knowledge and precision required to make a determination. The scale and nuance required by the problem make technological tools essential.
Two essential tools for CSAM detection
There are two primary tools that are available for programmatically detecting CSAM content: Hash matching and CSAM classifiers. Each of these tools serves a different purpose in the detection process. A process without one of the tools will have serious drawbacks and leaves itself vulnerable.
Hash matching for finding known CSAM
Cryptographic and perceptual hashing can be used to find previously identified CSAM images and videos that have been added to a hash list.
Cryptographic hashes are fast and small, but will only find byte-for-byte exact matches to a file. Perceptual hashing offers a solution that is resilient to minor modifications in the media and, for that reason, it is the primary tool used in the detection of known content.
Hash matching alone is an incomplete solution because it only detects images and videos that have previously been found and added to a hash list. To find new content, another approach is needed, such as a CSAM classifier.
The role of AI in finding novel CSAM
Predictive AI, specifically machine learning classification models, gives us the ability to find novel CSAM. The models use the underlying patterns that exist in CSAM to analyze and predict the likelihood that the content is CSAM. This is essential for uncovering new content, which is often of children experiencing active abuse. A CSAM classifier is the only tool that can identify novel images and videos at scale.
Facial age recognition + nudity detection ≠ CSAM classifier
Building an AI model to detect CSAM may seem simple at first. The naïve solution is to combine the predictions of models that can classify nudity and age in an image or video. At first glance, this seems like it solves the problem and could allow for the use of off-the-shelf models that have been heavily tested across different applications. Upon closer consideration, the drawbacks of this approach become clear.
Estimating age from a face
Most age estimation models require a face to be in the image. In fact, this technology is often referred to as facial age estimation. At Thorn, we know that many CSAM images do not contain the victim’s face.
Nudity needs more context
The presence of child nudity does not necessarily indicate that the content meets the legal definition of CSAM. A common example used to illustrate this is a family photo of a child in the bathtub. The content contains nudity but is not CSAM. At Thorn, we’ve specifically designed our training dataset to include benign images like this to improve the model’s accuracy.
A simplified model that predicts broad features in the content is not able to capture the nuance required in content moderation.
“It is important to note though that, while this method is not ideal, doing something suboptimal is far better than doing nothing when it comes to child safety,” said Michael Simpson, Staff Data Scientist at Thorn. “While some CSAM images do not contain a face for age estimation, some do, and those can be found using this method. Progress over perfection is one of our mantras here at Thorn and it unequivocally applies in this situation.”
The power of issue expertise
One key aspect to remember is that CSAM is not analogous to adult pornography. It is the documentation of children being abused. This leads to CSAM having several visual characteristics that make it unique. Thorn’s team knows about these differences thanks to our singular focus on this issue. We have gained critical insights from our own original research and our long relationships with law enforcement, hotline analysts, and content moderators. Additionally, our CSAM classifier was trained using data from trusted sources – in part using data from NCMEC’s CyberTipline.
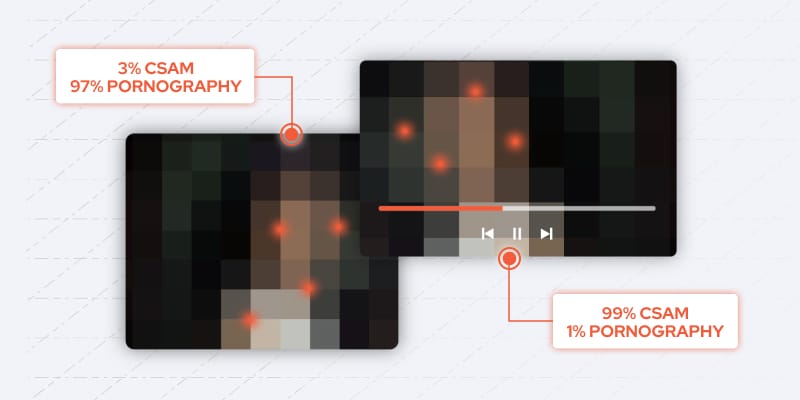
Understanding CSAM's unique characteristics
We use our knowledge to produce a more robust and accurate model. To illustrate, CSAM usually has these characteristics:
- A low production value
- Dirty and disorganized backgrounds
- Attempts to obscure the location where it was produced
These are all characteristics that the CSAM classification model is able to use to more accurately predict if content is CSAM.
Domain expertise also helps when it comes to removing patterns that should not be used. Early versions of the model would trigger when it noticed a large age discrepancy in an image containing a child and an adult, which is common in CSAM. But, this also occurs in many benign photographs (as mentioned earlier). We were able to address this by adjusting our dataset to include many examples of cases where an age discrepancy exists, but the image is not CSAM.
“We have spent years curating a comprehensive dataset that allows us to avoid common overgeneralizations in the AI model,” said Simpson.
Knowing there is a child behind every hash and data label
Thorn is an organization solely devoted to protecting children from sexual abuse and exploitation in the digital age. We work with NCMEC to use data with labels that have been verified by their world-class analysts. This data depicts some of the worst abuse imaginable. We always handle this data with respect and care as we work to stop future child sexual abuse.
Meeting different user needs
At its heart, all AI is pattern recognition. In order to build an effective model, a deep domain knowledge is essential. Thorn is able to use our knowledge of child safety to help weed out recurring mistakes that the classifier makes and focus the model on the patterns that make CSAM identifiable. The importance of this aspect is why, in the standard CRISP-DM modeling framework, the first two steps in the process are business understanding and data understanding.
Our direct involvement in this issue is why we are able to build an AI system that is trusted by many of the largest online platforms in the world. We take into account how our users will apply the model.
We understand that the tradeoffs between precision and recall are dramatically different for different applications. A large social network will have a very different base rate and tolerance for false positives than a law enforcement officer would have when looking for CSAM. By understanding this and designing around these constraints from the ground up, we are able to meet both of these users’ needs.
Meeting the moment: Generative AI and child safety
Generative AI poses an emerging threat to the child safety ecosystem. The traditional hashing approach is much less effective in an environment where a single individual can produce thousands of synthetic images in a few minutes. Thorn, along with All Tech is Human, has taken a lead role in addressing this problem by bringing together large AI companies to develop a set of principles and mitigations to prevent the misuse of generative AI to further sexual harms against children. The Safety by Design for Generative AI: Preventing Child Sexual Abuse initiative asks genAI companies to commit to a set of principles and agree to transparently publish and share documentation of their progress acting on those principles.
While traditional hashing struggles with the challenges generative AI poses, our CSAM classification model appears to remain effective in our internal testing. This sort of monitoring and analysis is why Thorn is able to stay ahead of emerging threats. By focusing our mission on a single harm, rather than dividing our attention across multiple harms, we are able to leverage our expertise to be proactive rather than reactive in the face of an ever evolving ecosystem.
Specialized tools make a difference
Emerging threats like AI-generated CSAM alongside the existing challenges of CSAM classification highlight the importance of purpose-built tools for trust and safety. Thorn is dedicated to equipping digital platforms with solutions and resources to address this issue head-on.
Continue exploring this series:
Introduction
Begin here.
What is CSAM? Common terms and definitions
Understand key terms and phrases.
Online child sexual abuse and exploitation statistics
Understand the scale of the issue.
Know the signs and risks of hosting CSAM
4 questions to help assess your platform’s risk.
Hash matching methods for CSAM detection
Understand the use case for available hashing methods.
CSAM Classifiers: Specialized detection methods
Understand the need for specialized CSAM detection.
Scene-sensitive video hashing for CSAM video detection
Understand how to detect CSAM within video content.
CSAM hash sharing across digital platforms
Learn about the available CSAM hash lists.